|
The
Parental Population
In our first attempt
at designing an evolutionary process,
we began with a parental population of genomes that were chosen
completely at random. We rapidly discovered, however, that randomly
assembled populations invariably evolved to a uniformly gray phenotype.
This undesirable property was a consequence of redundancies
in our developmental genetics. Because the location of a patch,
as well as its size, shape, and brightness, is specified as part
of the patch locus, our system could produce identical phenotypes
from very different genotypes. So breeding any two moths of identical
appearance could result in blending inheritance, producing on average a gray
moth, even in the absence of directional selection
(Kamil & Bond 2001).
To obtain biologically
realistic evolution, it was necessary to give the parental population a
selective history, making genomes that were structured by common descent
(Bond & Kamil 2002).
As a template, we chose a moderately cryptic moth image from an earlier
study (Moth #3 from
Bond & Kamil 1998)
and generated an initial population of fairly uniform appearance whose
phenotypes showed no more than a threshold level of disparity from the template
(in terms of taxonomic distance: Sneath & Sokal 1973). Genetic consistency
was obtained by subjecting this population to multiple generations of template-based
selection. At the beginning of each generation, the fitness of each
phenotype was determined by its discrepancy from the template moth.
Individuals were then selected to breed using a linear ranking algorithm
(Bäck 1996). Moths with the highest fitness scores had roughly
twice the probability of breeding as those with the lowest ones.
The selective regimen was maintained until the mean disparity from
the template, averaged over the entire population, was below a threshold
value, which generally required about 90-100 generations. This process
was repeated ten times, producing ten candidate populations with
moderate phenotypic variance and an internally consistent genetic
structure. The candidate population with the median level of phenotypic
variance was chosen to serve as the parental population for the
experimental runs. Below is a sample of moths from the parental
population on uniform (left) and cryptic (right) backgrounds.
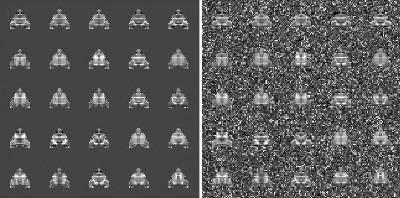
Reverse-Engineering
the Background
In these
studies, the moth stimuli were made hard to detect
by generating backgrounds that corresponded, to some specified degree,
to the distribution of pixel values in the moths. In essence, we
reverse-engineered the background, making the bark resemble the moths.
The degree of resemblance was controlled by creating random mixtures
of pixels drawn from two distributions: one that derived from
the moth stimuli themselves and a second, contrasting distribution that overlapped
minimally with that of the moths.
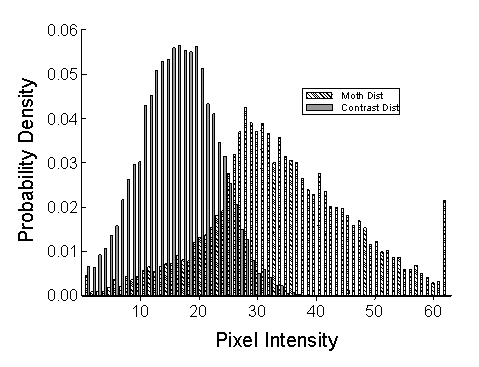
A background in
which 20% of the pixels were derived from the moth distribution
and 80% from the contrasting distribution would be referred to as
a Level 2 background, and the moths would be very easy even for humans to detect.
At the other extreme, finding the prey on a Level 8 background,
with 80% of the pixels drawn from the moth distribution, is exceedingly
difficult for all but a few highly proficient blue jays. The selection
experiments were all run with Level 3 or 4 backgrounds -- easy enough to
allow the birds to wipe out very conspicuous mutants, but hard enough
for hunting by searching image to produce a significant benefit.
After the background
pixels were selected, we adjusted the texture or "grain" of the image by randomly
adjusting the sizes of contiguous patches of the same gray-scale intensity level.
This was accomplished by a simple iterative process, in which the program
selected a pixel in the background at random and then changed it
to match one of its neighbors. The process was repeated many times,
until the distribution of patch sizes, measured with a flood-fiill
algorithm, matched the distribution derived from the moth phenotypes.
Measuring Crypticity
John Endler (1984,
1990) suggested that the level of crypticity could be quantified
by comparing the distributions of color patches in the organism
and the background. Since the patch sizes for our virtual moths
were constrained to mimic those of the background, the only variable
that influenced prey crypticity was the relative distribution of
pixel intensities. We were therefore able to develop a simple crypticity
measure based on the average unsigned difference between the pixel
distribution of a moth and that of the background.
This Crypticity Index varied from about 0.2 for the most conspicuous
moths to nearly 0.9 for the most cryptic ones.
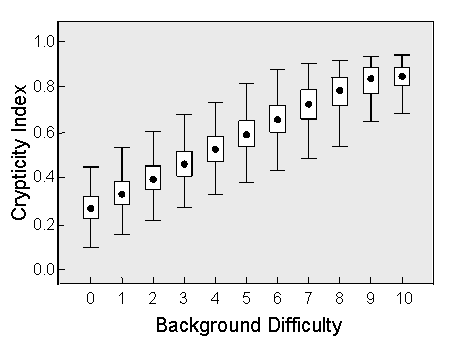
Evidence that the
index provided an adequate objective measure of background resemblance
came from two analyses. In the first, we took a single population of 200
moths (the
parental population for our study) and computed the distribution of
their crypticity indices over the full range of background levels.
As shown above, the results are approximately linear, as one would
expect from the fact that each successively higher level of background
difficulty is produced by increasing the number of moth-derived pixels
by a constant percentage. The crypticity index thus faithfully
reflected our algorithm for generating matching backgrounds.
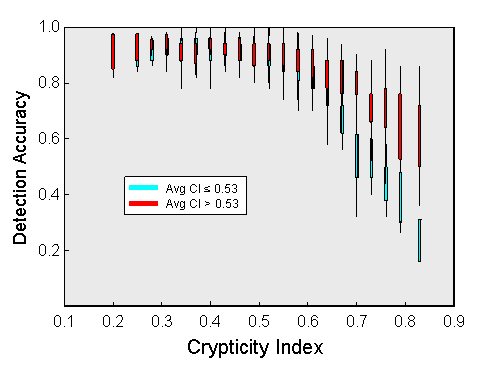
Secondly,
during the experimental trials, the crypticity index accounted for better
than one-third of the variance in the detection accuracy of the birds.
Although jays found most of the more conspicuous moths (with indices
of less than 0.5), their performance declined rapidly with higher
index values. The effectiveness of a particular level of crypticity depended,
to some degree, on the average crypticity index for the entire population.
Highly cryptic individuals derived less benefit in populations where
most of the other moths were also cryptic (shown in red above) than
in populations that were generally more conspicuous (shown in cyan).
This is a reflection of a "search rate" (Guilford & Dawkins 1987)
or "caution"
(Bond & Riley 1991)
effect. When the birds expect
a difficult detection task, they search each display more persistently,
increasing their chances of detecting a cryptic individual.
References from Other Sources
Bäck, T. (1996). Evolutionary Algorithms in Theory and Practice. New York:
Oxford U. Press.
Endler, J.A. (1984). Progressive background matching in moths, and a quantitative measure of
crypsis. Biological Journal of the Linnean Society 22: 187-231.
Endler, J.A. (1990). On the measurement and classification of colour in studies of animal
colour patterns. Biological Journal of the Linnean Society 41: 315-352.
Guilford, T, & Dawkins, M.S. (1987). Search images not proven: A reappraisal of
recent evidence. Animal Behaviour 35: 1838-1845.
Sneath, P.H.A., & Sokal, R.R. (1973). Numerical Taxonomy: The Principles and
Practice of Numerical Classification. San Francisco: Freeman & Co.
|